Self-Replication, Spontaneous Mutations, and Exponential Genetic Drift in Neural Cellular Automata
This paper reports on patterns exhibiting self-replication with spontaneous, inheritable mutations and exponential genetic drift in Neural Cellular Automata. Despite the models not being explicitly trained for mutation or inheritability, the descendant patterns exponentially drift away from ancestral patterns, even when the automaton is deterministic. While this is far from being the first instance of evolutionary dynamics in a cellular automaton, it is the first to do so by exploiting the power and convenience of Neural Cellular Automata, arguably increasing the space of variations and the opportunity for Open Ended Evolution.
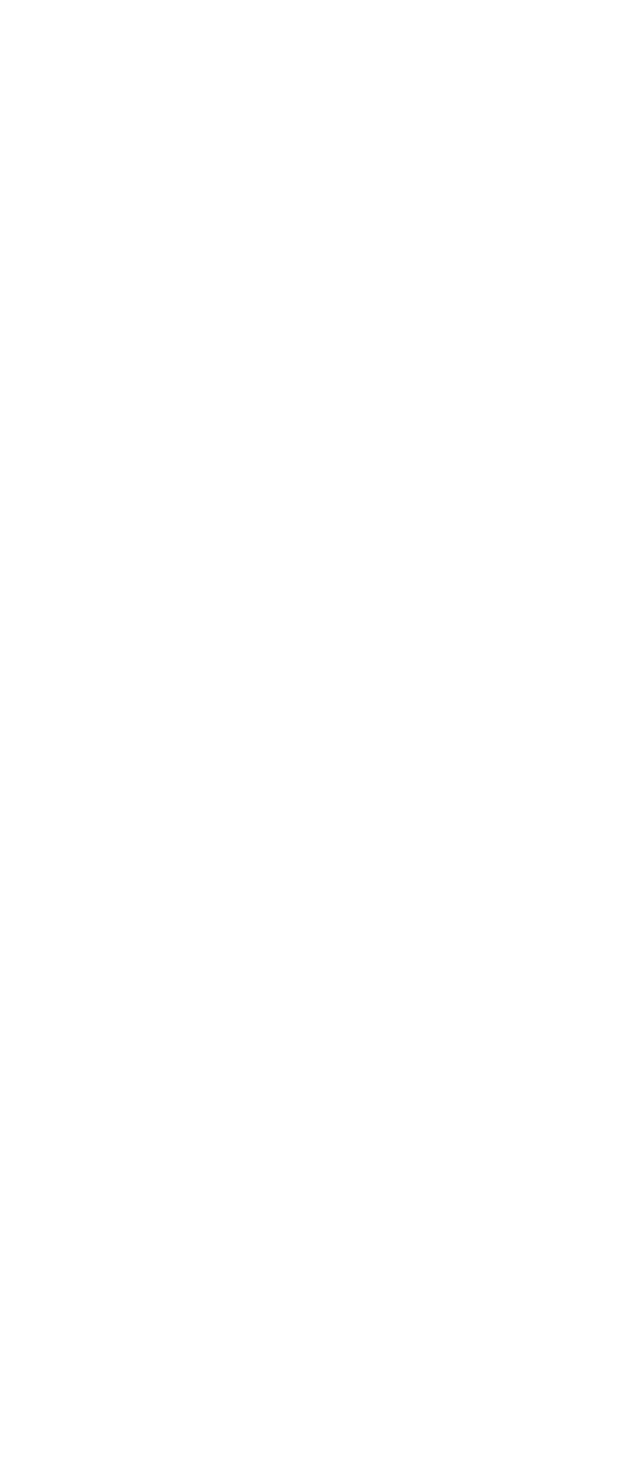
Figure 2:Simple self-replication. (a) Results shown for a NCA is trained to self-replicate from a bacteria emoji. (b) To analyze the successive generations without interference from the grid becoming crowded with bacteria, we isolate one bacteria after replication (top or bottom, chosen randomly) and transplant it to a blank grid where it can replicate again. (c) Note the visual differences (mutations) between successive generations: G5 seems to have 2 nuclei (yellow central patch). Mutations extends to the non-RGBA values of each cell's state. Grid lines are not shown, but each pixel is a distinct cell of the automaton.
Figure 3:Growth and self-replication. We add a self-replication step to the growth phase first introduced by Mordvintsev et al., 2020. (a) The NCA is trained for 1000 training steps by alternating between an intermediary target (grow fish from egg) and a final target (move fish left and lay egg), as can be seen in the divided loss curve (b). (c) Successive generations show signs of mutation: by generation 98 the fish has lost one of the target's 3 stripes, but generation 99 regains it and generation 100 adds a supernumerary 4th stripe.
Figure 4:Genetic coding and drift. A different run of the model in Figure 3, at training step 1500. (a)~MSE between the fish at generation 0 and its descendants. The descendants appear to be all equally different from the 0th generation, except for a jump at generation 82 where the fish develop a forked stripe that is inherited by successive generations. (b)~When calculating genetic drift, we find not a linear relationship as in~(a), but an exponential increase in MSE until generation 82, where this model stalls (not all models stall in 100 generations). (c)~The clear correlation indicates the emergence of a genetic code: DNA differences in the eggs are translated to phenotype differences in the developed organism, and big DNA mutations correspond (mostly) linearly to big phenotype differences.
Figure 5:Exponential drift in a 2-organism NCA. (a) The model is trained to develop different DNA into 2 different patterns, as well as replicating the patterns. (b) In some cases, especially before training convergence, the model goes back and forth between fish, lizard, and hybrids in the same lineage. (c) In this other lineage the DNA undergoes a relatively smooth transition, while the phenotype abruptly switches from fish to lizard. (d) The phenotype space is large, and the average difference of DNA (genetic drift) and phenotype (phenotypic drift) between an ancestor and its descendants increases exponentially.
These mutations despite the NCA rules being deterministic could be due to rounding errors that often occur with floating point number representation. It is also possible that stochasticity is introduced elsewhere in the model unbeknownst to the experimenter, or due to the equipment (stochasticity in GPU runs). Note that these causes would still satisfy the definition of closed model by (cite: OEEprize). Finally, there is the possibility that each division is inherently different from the previous one, i.e. that the model that is genuinely deterministic but chaotic. This last hypothesis is reinforced by the fact that running the synchronous model from the same starting point always seems to lead to similar final results, even if those results are far from the initial state.
- Mordvintsev, A., Randazzo, E., Niklasson, E., & Levin, M. (2020). Growing Neural Cellular Automata. Distill, 5(2). 10.23915/distill.00023